What is Allora Network?
With the rise of AI and Big data, significant advances have been made in the realms of data access and computing, making machine intelligence capable of delivering valuable insights. However, the vast resources required for these systems mean they are often controlled by large companies and kept private. To unlock the true potential of machine intelligence, data, algorithms, and users must be fully connected. Blockchain technology, with its decentralised and open-source nature, is the perfect solution to this challenge.
Introducing Allora – a decentralised machine intelligence network designed to democratize access to AI models and computation. It allows users to contribute and utilize AI resources without relying on centralised entities, enabling collaboration and innovation in AI-driven applications. By leveraging decentralised infrastructure (i.e. Blockchain), Allora promotes scalability, security, and open access, making AI tools more widely available across various industries.
For the rest of the article, we take one step back to build an understanding of the core technologies behind Allora, before presenting a high-level overview of how it operates.
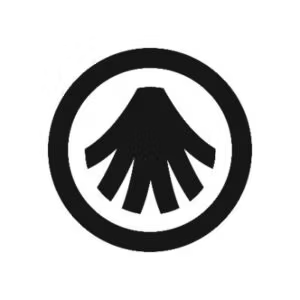
What exactly is Machine Intelligence?
Machine intelligence refers to the accuracy of outputs generated by algorithms, whether they rely on machine learning (ML), deep learning (DL), or traditional methods. It’s a broad concept used to describe how effectively these algorithms produce valuable results. In the business-to-business (B2B) tech sector, the term also refers to a growing market for companies that offer machine learning and artificial intelligence services, commonly known as MLaaS (Machine Learning as a Service) and AIaaS (Artificial Intelligence as a Service).
Machine intelligence, in this context, represents a machine’s ability to learn from data and proactively make decisions. By gathering and analysing different types of information, machines can develop their own processes and reach conclusions, integrating the capabilities of both machine learning and artificial intelligence.
What Problem is Allora trying to solve?
The problem Allora addresses goes beyond simply siloed machine intelligence; it deals with the structural limitations of current machine intelligence development and usage. Presently, machine intelligence is dominated by industry giants with exclusive control over vast datasets, sophisticated algorithms, and computational power. Such concentration of resources creates several core issues:
The monopolisation of Resources: Large companies hold exclusive control over the most advanced AI and machine learning systems, making it nearly impossible for smaller players to access the same level of intelligence, leading to a lack of competition and innovation.
Lack of Transparency and Openness: Because these machine intelligence systems are siloed within organizations, there is little transparency around how algorithms work, how data is used, or how decisions are made. Such a lack of openness prevents collaboration across different organizations, limiting the development machine intelligence.
Barriers to Entry: The high costs of acquiring data, designing algorithms, and investing in computational infrastructure—mean that only well-funded entities can participate. Therefore, making machine intelligence a highly exclusive domain via creating a significant barrier for developers, startups, and smaller companies to enter and contribute.
Suboptimal Incentive Structures: Existing networks reward participants based on historical reputation or other static factors, rather than dynamically adjusting rewards based on the context and the specific tasks being performed. Hence, a lack of context-aware intelligence limits the effectiveness of these systems.
To solve the above issues, Allora has put together a strong team of AI/ML & Blockchain experts and raised $35 million in total venture investment to bring their idea to market. Read on as we demonstrate how Allora is improving AI & ML industry.
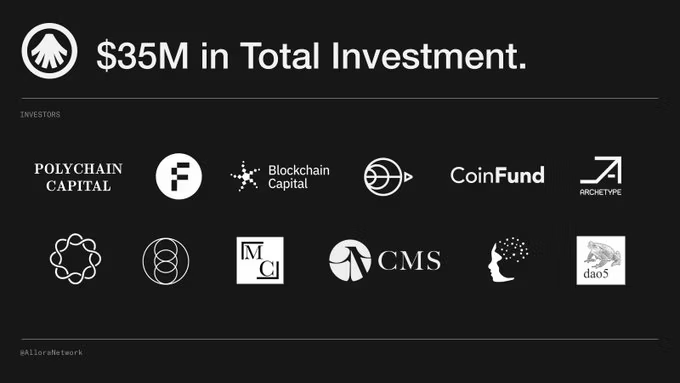
(Source: @AlloraLabsHQ, X)
How is Allora addressing the consequences of soiled intelligence?
Allora solves the issues caused by siloed machine intelligence via creating a decentralized network that enables open collaboration between data providers, AI models, and end users, while also offering a system that continually improves over time. Below we offer a more detailed breakdown of Allora’s solutions.
Allora’s Architecture
The Allora network functions through three main parts:
- Inference Consumption Layer: This is where information is exchanged. Users access insights or “inferences” made by the system.
- Forecasting and Synthesis Layer: Here, two types of workers play a role:
1. Inference workers provide the initial insights
2.Forecasting workers predict how accurate these insights are using models. Then, the system brings all the results together for users - Consensus Layer: This part handles the system’s rewards and overall operations, ensuring that everything runs smoothly and securely without needing permission.
You can see a visual representation of Allora’s architecture below:
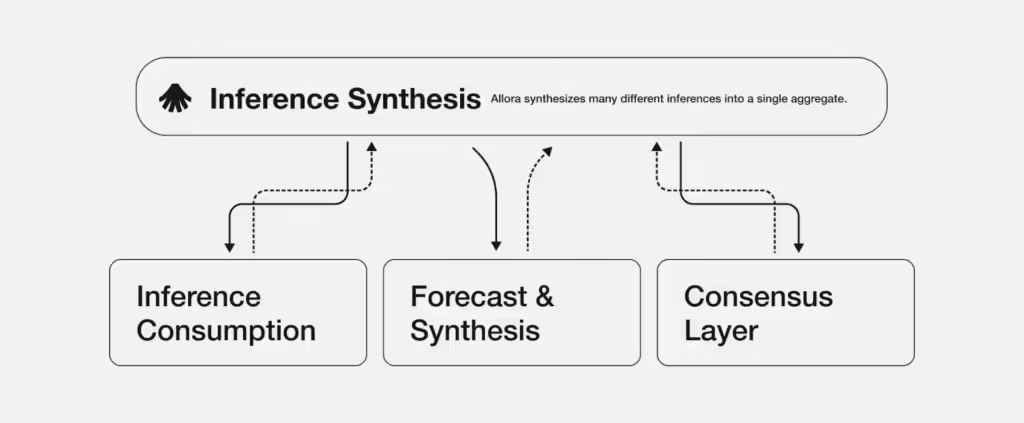
(Source: Allora Docs)
Transparency & Collective Intelligence
Instead of large companies controlling machine intelligence in isolation, Allora uses a peer-to-peer network where AI agents generate and share their predictions, called inferences. Open access makes AI more accessible to everyone, removing the control that industry monoliths have over data and algorithms. Allora allows data owners, processors, and end users to work together seamlessly, which fills the silos that exist in traditional machine intelligence.
Additionally, Allora creates a network where AI agents, called workers, generate inferences and forecasts. These are then evaluated by other participants, called reputers, using a consensus mechanism to ensure transparency, as every inference is evaluated openly by the network. All contributions are visible and judged fairly, which promotes collaboration between participants, unlike the closed systems of large companies.
Overall, Allora enhances accuracy by combining inferences from multiple participants using context-aware forecasting, where workers predict the accuracy of others’ inferences. As a result, the collective approach yields better insights than individual AI models.
Incentives & Sub-Networks
To encourage participation, Allora has a unique incentive structure that rewards participants based on the value they bring to the network. Workers who provide accurate inferences or help forecast performance receive rewards proportional to their contribution. Reputers, who assess the quality of inferences, are also rewarded based on the correctness of their evaluations. These rewards ensure that each participant is motivated to improve the network, making it continually smarter and more effective over time.
Besides tailored rewards, Allora introduces “topics,” which are smaller sub-networks that focus on specific problems or areas. These topics allow participants to collaborate within defined rules and focus on optimising a particular target, creating a flexible structure that addresses different needs. Such a modular approach ensures that the network can solve a wide range of problems, depending on the topic being addressed, making the system versatile and adaptable.
Finishing the puzzle…
Allora’s solution comes down to the harmony between 3 key ecosystem players including: Topic coordinators, workers and reporters to output a self-improving, decentralised machine intelligence network. Below we explain the connection between hey players:
- Topic Coordinators are responsible for defining the rules, setting the goals, and coordinating AI tasks within the network. They manage requests from users who seek AI-powered insights, distributing tasks among the Workers while also tracking predictions from Reputers who assess the quality of the output.
- Workers are responsible for generating the AI inferences (predictions or insights) based on the tasks set by the Topic Coordinator. They also forecast the performance of inferences generated by other workers. This adds a layer of validation by cross-checking the expected quality of outputs from different perspectives.
- Reuters score the inferences against ground truth (the correct or most accurate outcome). Based on these evaluations, they provide feedback to the Topic Coordinator, ensuring that the quality of the inferences is continually refined.
On a high level, the above players interest on 4 key steps:
- User Interaction: A user makes a request for AI-driven insight through the Topic Coordinator.
- Task Assignment: The Topic Coordinator assigns this request to Workers, who generate inferences. It also assigns forecasting tasks where Workers predict the likely success of their peers’ work.
- Feedback Loop: Once the Workers complete their inferences, Reputers evaluate these inferences by comparing them to the ground truth. They then send this scoring data back to the Topic Coordinator.
- Network Inference: The system continually adjusts its forecasts and improves based on both the inferences generated by Workers and the scoring from Reputers, optimizing future performance through context-aware adjustments.
The Diagram below illustrates the interaction between key players:
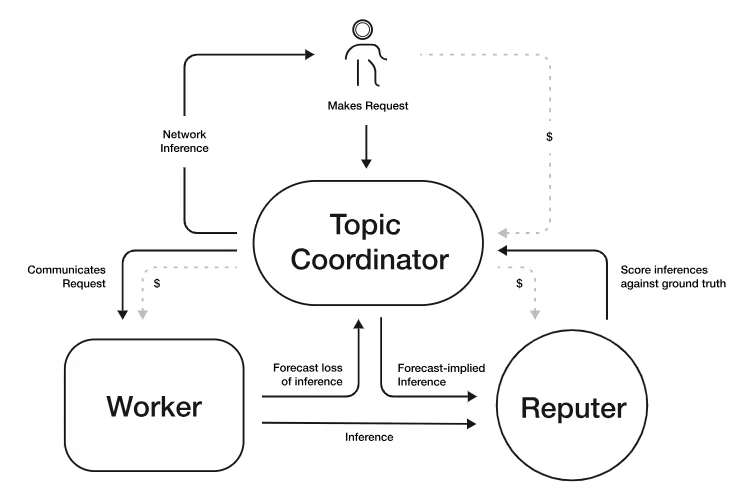
Currently, Allora is on Testnet, meaning that the network is being tested in an environment that mimics publicly available blokchains. Simply is honoured to be an early supporter of Allora! You can delegate your coins to us via our Networks Page!
Applying Allora to the real world
By now, you can imagine the number of real-world applications that Allora could have, running from predicting sports outcomes to election results and testing code pre-deployment. Let’s apply allora to climate forecasting!
A government or research organization requests AI-driven climate insights, such as predicting weather patterns for a specific region. The Topic Coordinator sets the goals and breaks down the forecasting tasks. These tasks are assigned to Workers, who use their AI models to generate predictions, such as temperature trends or precipitation levels. Workers also predict how accurate the forecasts of other Workers will be, adding a validation layer.
After the predictions are made, Reputers compare the forecasts to actual weather data (ground truth) and evaluate how close the predictions were. This feedback is sent back to the Topic Coordinator, which adjusts future predictions by refining the AI models based on the performance evaluations.
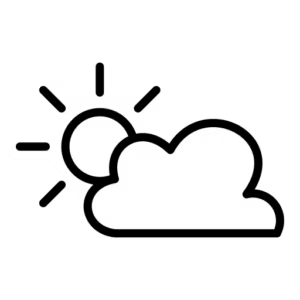
Through this collective process, the network generates more accurate and refined weather forecasts than what a single AI system could achieve on its own.
Economics of Allora
Allora couples sustainable machine learning with an equally sustainable economic model to foster a long-term, stable growth of it’s ecosystem. To this end, allows has introduced a Pay-What-You-Want business model together with the ALLO token. Stick with us as we explain both of these below!
Pay-What-You-Want (PWYW)
The Pay-What-You-Want (PWYW) model in the Allora Network offers token holders the freedom to determine how much they pay in ALLO tokens for inferences generated by the network. The PWYW creates a more inclusive and accessible environment by allowing participants to assign their own value to the service, rather than having fixed fees.
Moreover, token holders can choose any amount to pay, which encourages a personalized valuation of the network’s output. However, if participants opt to pay zero for a particular topic, that topic will be deprioritized, and its weight in the network will be reduced to zero, meaning that workers within the deprioritised valuation won’t receive rewards, and token emissions will be reallocated to other, more active topics.
Overall, the PWYW structure promotes healthy competition across topics and allows the market to naturally negotiate a fair price. Over time, price discovery occurs as participants determine the value of different topics, ensuring that only valuable and well-supported topics continue to grow.
The ALLO Token
ALLO tokens are native assets on the Allora Network and serve use-cases beyond purchasing the inferences. ALLO tokens are required for creating or participating in topics, where Workers and reputers need to pay a registration fee in ALLO to engage with specific topics, contributing to the network’s ecosystem.
Staking plays a significant role, as reputers and network validators use ALLO tokens for staking, while token holders can delegate their stake to these participants. Both staking and delegation are rewarded, with rewards distributed in ALLO tokens to reputers, validators, and delegating token holders.
The rewards in ALLO are distributed in the following way:
- Workers are rewarded based on the quality of their inferences
- Reputers are rewarded based on the accuracy of their evaluations and their stake in the network
- Network Validators are rewarded solely based on their stake in the network
Keep an eye out on our blog, as we will be publishing a more technical piece that dives into tokenomics more deeply!
Talking about tokens, you can buy your crypto through our partnership link with Binance and get $600!
(*Terms & Conditions Apply)
Conclusion
In conclusion, Allora Network offers a decentralized solution to the structural limitations of centralized machine intelligence systems. By facilitating open collaboration between data providers, AI models, and users, Allora promotes transparency, collective intelligence, and tailored incentives that reward participants based on their contributions. Its features, such as context-aware forecasting and sub-networks for specialized tasks, enable continuous improvement and adaptability. Coupled with an economic model centred on the ALLO token, Allora creates an inclusive environment that allows a wide range of participants to contribute to and benefit from advancements in machine intelligence.
You can simply stake and secure your assets with the Ledger wallet.
Disclaimer: This article contains affiliate links. If you click on these links and make a purchase, we may receive a small commission at no additional cost to you. These commissions help support our work and allow us to continue providing valuable content. Thank you for your support!
This article is provided for informational purposes only and is not intended as investment advice. Investing in cryptocurrencies carries significant risks and is highly speculative. The opinions and analyses presented do not reflect the official stance of any company or entity. We strongly advise consulting with a qualified financial professional before making any investment decisions. The author and publisher assume no liability for any actions taken based on the content of this article. Always conduct your own due diligence before investing.